How to track SERP History Without the Hassle and Bank Breaking

Understanding how website rankings, competitor activity, and other SEO-related factors change over time is crucial for any SEO strategy. By analyzing SERP history data, you can gain valuable insights into the factors impacting your website’s visibility in search results. Additionally, incorporating SERP snapshots allows for a more complete view of these changes, as you can visually capture and analyze how the SERP appeared at the precise moments you make your queries.
In this article, we will review tools that can be used to track Google SERP history. We will also discuss creating your tool to collect the necessary data. We also will provide a script that anyone can use to collect SERP history.
Tools for SERP History Collection
SERP history collection tools are designed to track and store changes in search engine results pages (SERPs). Using these tools, you can analyse the dynamics of changes in website rankings, keywords, and other parameters in the SERP.
Get real-time access to Google search results, structured data, and more with our powerful SERP API. Streamline your development process with easy integration of our API. Start your free trial now!
Gain instant access to a wealth of business data on Google Maps, effortlessly extracting vital information like location, operating hours, reviews, and more in HTML or JSON format.
There are two main approaches to collecting SERP history: saving data about the SERP or saving a snapshot of the SERP. The latter approach is more convenient and provides more complete data, allowing you to view the SERP as it appeared at a given time.
What is a SERP Snapshot?
A SERP snapshot is a visual representation capturing the precise state of a search engine results page at the moment a query is executed. This snapshot provides valuable information into the specific composition of the SERP, including the positions of various web pages, featured snippets, and other rich elements.
There are several different tools available for SERP snapshots:
SEO tools and platforms. Many SEO platforms, such as Serpstat, Ahrefs, and SEMrush, offer SERP tracking tools. These tools typically provide data on website rankings, competitor activity, and other metrics. However, they can be expensive, pricing upwards of $200 per month. Additionally, some tools provide limited information, such as website rankings and links.
SERP APIs. Some services offer APIs that can be used to collect SERP data. This approach is more flexible and affordable than using an SEO platform. APIs typically provide all available information from the SERP, including snapshots, and you can choose what data you need. Additionally, API-based data collection tools can be integrated into your applications.
Web scraping services. You can also use web scrapers to collect SERP data. Web scrapers are tools that can automatically extract data from websites. This approach is the easiest way to get the necessary data.
The best option for collecting SERP history is to use an API that can provide data about search results and a page snapshot. This will allow you to view exactly how the search results looked at any given time. As an example, we will use HasData SERP API, which returns this data.
How to Track Historical SERPs
This tutorial will show you how to track SERP history using a SERP API, suitable for non-programmers and those who want to create their script. The easiest way to get the data is to use a pre-configured Google Sheet with App Script.
If you want to create your flexible tool without getting into programming, use Make.com or Zapier to create simple integrations. Or, you can create a script that will perform your needed functions.
Use Google Sheets Template
The easiest way to collect Google search results snapshots is to use a ready-made template. To do this, copy our Google Spreadsheet and launch the HasData add-on. Select Rank Checker, and enter your API key, keywords, and a link to the domain you want to check the position. You can also customise the other parameters as desired.
Once you have configured Rank Checker, you can launch it to get snapshots and links to the found page for every keyword, along with its current position. To collect an archive of search results screenshots, simply run Rank Checker at the desired frequency.
In addition to collecting snapshots and tracking positions, you can use other features of this template, such as automatic position tracking with Google Sheets. For more information, see our article on Rank Tracker in Google Sheets.
Use Zapier or Make.com Integration Service
Another solution is to use integration services to create personalised apps without programming skills. Zapier and Make.com are the most popular services of this type. We have already covered how to develop zaps with Zapier, get data from Google SERP, and various websites with Make.com.
To create a SERP history tracker in Zapier, create a trigger for the snapshot collection, the keyword source, and the HasData SERP API. This will result in the following data:
You can then integrate this data with Google Sheets, email, or another service to save or process it.
Make.com has more flexibility for processing arrays. For periodic SERP history snapshots scraping for all keywords, you can use Make.com’s capabilities without adding additional sources. The rest of the approach is the same as for Zapier.
You can also decide how to process or store the collected data and integrate it into your app.
Use Python Script
To get started, we have provided a ready-to-run script on Google Colaboratory so you can use it immediately. You’ll need to enter your API key and keywords to do this. Once you’ve done that, you can run the script to get the data.
The Google SERP API library for Python is a comprehensive solution that allows developers to integrate Google Search Engine Results Page (SERP) data. It provides a simplified way to get organic search results, snippets, knowledge graph data, and other data from the Google search engine.
This easy-to-use interface lets you call the Google SERP API to efficiently scrape search engine results (SERPs) using Node.js. It simplifies retrieval of organic search results, snippets, knowledge graph data, and more from Google.
If you want to make your own, create a new file with the *.py extension and import the necessary libraries. In this script, we will use:
The Requests library to make HTTP requests to the API.
The OS library can check the file where we will store the SERP history data.
The DateTime library adds the current date and time.
The Pandas library creates a data frame and saves the data to a file. In this guide, we will save the data to a CSV file, but Pandas also supports other file formats.
All libraries except pandas are pre-installed and can be used immediately, and to install pandas, you can use the command:
pip install pandas
To import the libraries to your Python script, use the following code:
import requests
import pandas as pd
from datetime import datetime
import os
Now, let’s define the variables we will store the data. We need two - one for storing the processed keywords and one for collecting the results. We will use them later:
keywords = []
results = []
Set the variable in which you can place the keywords that will be processed in the future and added to the keywords variable:
serp_keywords = ["""
web scraping, top scraping tools
scraper
"""]
This approach is needed for convenience, as it is sometimes easier to specify keywords with a new line and sometimes with a comma. Therefore, to avoid errors in the future, we will first place the original data in a separate variable and then process it and create an array.
Next, we will set the parameters for working with the API - the link, the API key, and the basic parameters, such as the Google domain, the country, and the search language. This is necessary to make the request more customised and useful:
api_url = 'https://api.hasdata.com/scrape/google'
headers = {'x-api-key': 'API-KEY'}
base_params = {
'domain': 'google.com',
'gl': 'us',
'hl': 'en'
}
To continue, we need to extract all keywords from the string stored in the serp_keywords variable. To do this, we will split it line by line and check for commas as a separator. After that, we will add each keyword to the keywords variable:
serp_keywords = [line.strip() for line in serp_keywords[0].split('\n') if line.strip()]
for input_line in serp_keywords:
words = input_line.split(',')
if words:
keywords.extend(words)
Now, we can iterate over the resulting array element by element and collect snapshots for each query. Let’s add a loop to iterate over all keywords and add it as a parameter for calling the API along with the basic parameters added earlier.
for keyword in keywords:
if keyword.strip():
params = {**base_params, 'q': keyword.strip()}
try:
# Here will be code
except Exception as e:
print('Error:', e)
We used a try/except block to isolate code from errors that could occur during the execution of requests. With this approach, even if an error occurs when processing a keyword, the script will output error information to the console and move on to the next keyword instead of completely halting execution.
Make an API request, and if the response is positive (status code 200), store the keyword, Google SERP snapshot URL, and current date and time in the results variable:
response = requests.get(api_url, params=params, headers=headers)
if response.status_code == 200:
data = response.json()
print(f"{keyword.strip()},{data['requestMetadata']['googleHtmlFile']},{datetime.now().strftime('%Y-%m-%d %H:%M:%S')}")
result = {
'Keyword': keyword.strip(),
'Screenshot': data['requestMetadata']['googleHtmlFile'],
'Date': datetime.now().strftime("%Y-%m-%d %H:%M:%S")
}
results.append(result)
Finally, after iterating over all keywords, let’s save the historical data to a CSV file. We will check if the file exists, and if not, we will create it. If the file does exist, we will simply append the data to the existing file.
df = pd.DataFrame(results)
csv_file_path = 'serp_history.csv'
file_exists = os.path.isfile(csv_file_path)
mode = 'a' if file_exists else 'w'
df.to_csv(csv_file_path, mode=mode, index=False, encoding='utf-8', header=not file_exists)
Let’s execute the script step-by-step and check the results.
As we mentioned earlier, the whole script is available in Google Collaboratory.
Conclusion
In this article, we have explored the different ways to collect SERP history, including SEO platforms and APIs. SEO platforms offer a wealth of data, but APIs provide flexibility and cost-effectiveness, especially for specific needs.
The provided Python script provides a practical example of using the HasData SERP API to gather and analyse SERP ranking history data. This script captures keyword snapshots and timestamps and exports the information to a CSV file for in-depth analysis.
By leveraging SERP history tools, you can gain valuable insights into search engine rankings, competitor activity, and other SEO-related factors. This data can inform your SEO strategies and elevate your website’s visibility in search results.
Might Be Interesting
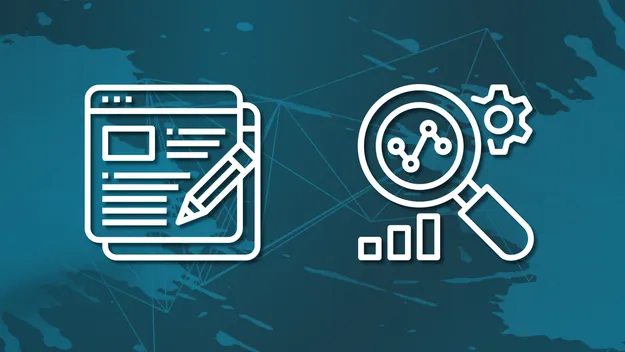
Dec 19, 2024
How to Do Your Own Content Gap Analysis
Improve your content with an easy gap check. Find what you lack, fill it, and make your site more valuable to you and your audience.
- SEO Tools
- Use Cases

Dec 6, 2024
XPath vs CSS Selectors: Pick Your Best Tool
Explore the key differences between CSS selectors and XPath, comparing their advantages, limitations, and use cases. Learn about performance, syntax, flexibility, and how to test and build selectors for web development.
- Basics
- Use Cases
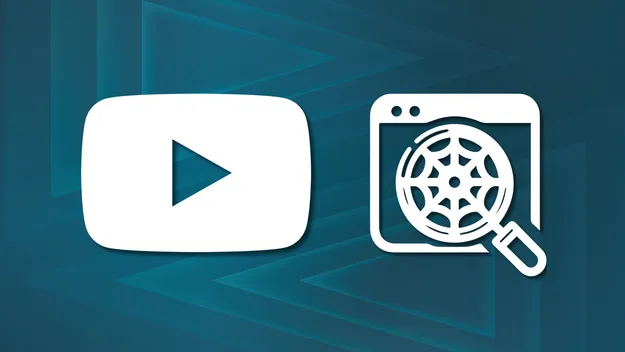
Oct 29, 2024
How to Scrape YouTube Data for Free: A Complete Guide
Learn effective methods for scraping YouTube data, including extracting video details, channel info, playlists, comments, and search results. Explore tools like YouTube Data API, yt-dlp, and Selenium for a step-by-step guide to accessing valuable YouTube insights.
- Python
- Tutorials and guides
- Tools and Libraries